The understated power of simple investment decisions
Investment portfolios represent the culmination of several decisions. The quality of these decisions varies, and it is important to align your risk exposure accordingly. Decisions based on highly sophisticated analysis in complex situations can be impressive and, if they should turn out to be profitable, can even confer a belief that an investment manager has significant insight. However, we outline the significant hindrances to producing an accurate prediction in complex situations and contrast this with some examples of simpler decisions. A key element of risk management is calibrating exposure to insights after taking their accuracy into account.
Identifying complex decisions
Complex investment decisions can be identified by observing their underlying components. Some indicators which point to the presence of a complex decision include:
- A critical reliance on a relationship between variables which is not a straight line;
- Arguments being made which rely on a chain of inference with many links;
- Relying on forecasts of variables that cannot be reliably measured;
- Attempts to forecast the outcome of ‘reaction functions’ which are not well understood or unstable; and
- The presence of adaptive processes, where the situation consistently evolves to ensure that forecasting remains hard. For example, there are other motivated competitors who are learning quickly to erode any intellectual advantage you may have temporarily developed.
Economic forecasting is notoriously difficult, and the magnitude of the revisions to expectations that have taken place in the last six months has been significant. Over this period, we have seen:
- Conviction in the idea of transitory inflation arising from temporary supply chain difficulties disappear;
- The RBA abandon the management of the yield on the Australian Commonwealth Government April 2024 bond (a captain’s call by Phil Lowe ahead of the November 2021 RBA meeting) and the progressive loss of ‘patience’ which culminated in a surprisingly large rate rise in early May;
- War erupt in Ukraine which created large spikes in the price of energy and grains, contributing to multi-decade highs in consumer inflation readings;
- Strong demand for labour which, in the case of Australia, has resulted in an unemployment rate that is close to the lowest recorded in the last 50 years;
- An Omicron wave which was dealt with via a significant shift away from mandated lock-downs towards a higher emphasis on personal responsibility;
- Large floods on our east coast; and
- The timeline and pathway for the reduction in central bank balance sheets move from a possibility that could be considered for late 2023 and be relatively measured, towards a debate on the pace of imminent reduction that will not cause market dysfunction.
A lot happens in six months! Along the way, the market inferred expectations for the RBA Cash rate as at December 2022 rose from 0.1% to around 3% and left the great rump of economic forecasters trailing market pricing by some margin as this developed. Even the RBA was compelled to acknowledge the magnitude of their own forecasting errors. There is never a shortage of opinions on the future path of cash rates but how much should you rely on them?
The brief list of significant events in recent months, whose occurrence and impact are very hard to predict with any accuracy at all, has dominated the movement of expected rates. If these elements are collectively close to unpredictable, even incredibly insightful economic forecasters would have a hard time producing high-quality predictions that should meaningfully influence portfolio outcomes, especially for medium-term tactical positioning.
An example of a complex decision
For these reasons, macro forecasting has a limited role when forecasting absolute cash rates over the next year or two because extraneous and very influential events tend to occur over this time frame. However, even if large external shocks did not occur and we restricted ourselves to the standard type of macroeconomic analysis, forecasting the future path of the cash rate would remain extremely hard.
In this section, we will consider the difficulty of the challenge of applying traditional macro-economic approaches to forecasting cash rates.
We will highlight characteristics of the forecasting challenge which appear on our incomplete list of indicators for a complex decision. In doing so, we aim to highlight that forecasting in this manner encounters very high levels of irreducible uncertainty and this keeps forecasting ability inherently low. We present this example as an illustration of a complex decision rather than a targeted comment on cash rate forecasts using macro-economic analysis.
Presence of a non-linear relationship: One of the key elements of the RBA’s position on rates is the outlook for wages. Wages growth is assumed to follow a relationship called a Phillips Curve, where wage growth is inversely related to a measure of tightness in the labour market:
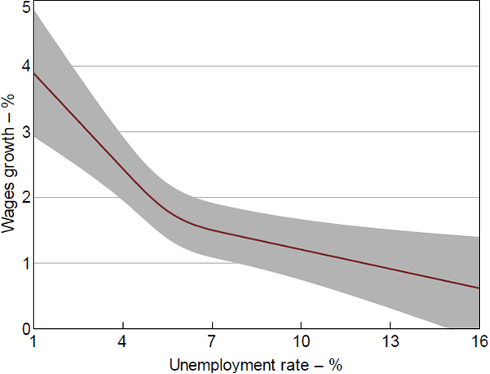
Whilst the concept of an inverse relationship makes sense, the accuracy of the estimated relationship is very low for practical purposes. Indeed, the RBA and Treasury both explain that we have very limited recent experience of the behaviour of wages under the current level of labour constraint.
The assumed point at which the curve bends materially is closely related to the NAIRU (non-accelerating rate of unemployment). However, relatively limited changes in assumptions relating to this figure can produce very significant differences in the outlook for wages. The recent Budget documents show the impact of different plausible alternatives to the best estimate of a NAIRU of 4.25%. These alternatives produced a range of wage outcomes that infer anything from holding rates low for years or imminently launching into a series of rate hikes:
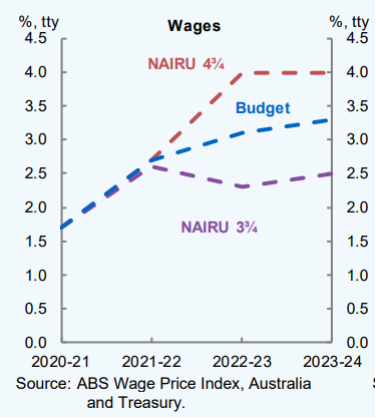
This uncertainty was one of the reasons why the RBA had previously placed significant emphasis on observing wage outcomes and not relying on predictions before moving rates. So, even if you were able to produce a fully accurate forecast for unemployment and could measure wages reliably, the ability to forecast wages growth is very impaired because the relationship between them is not particularly well understood. Further, if collective bargaining processes were to become very established again, or the psychology of wage-setting were to evolve from its recent history where a focus on cost containment has been prevalent, the relationship will keep shifting. These issues make accurate estimates of the relationship difficult to attain via these methods because it is inherently unstable.
Reliance on a chain of inference: Even if the relationship between economic or financial variables is well understood, if there is a chain of inference, the power of any forecast linking the first to the final step in the chain can be strongly eroded. For example, imagine a statement that nests a string of statements:
- I expect unemployment to surprise to the upside;
- This will lead to wages surprising to the downside; and
- As a result, the RBA will tighten rates later than the market currently expects.
As we know, economic forecasting is hard, but let’s assume this is the work of a forecaster with an exaggerated level of foresight which we will represent with a 75% accuracy rate at each point. This is very unlikely to be true for an insight of this type. After all, as we have observed above, modest moves in unemployment do not have a particularly accurate relationship with wages so moderately higher unemployment does not necessarily lead to lower wages. Further, the RBA’s statements on the influence of wages for rate-setting have been…wobbly. Nonetheless, if the forecaster is 75% accurate at each of the three decision gates, the ability to predict cash rates better than the market, arising initially from an insight on the level of unemployment, improves from 50% to 56.3%. That is a useful margin.
However, when it comes to economics and links to most financial variables, sustained 75% accuracy rates on calls of this type are exceedingly rare. Even a 60% accuracy rate would be excellent.
Following the same chain of inference with a 60% accuracy rate would only produce a 50.4% accuracy through to the final step of the forecast. This is very close to random and would not be useful for portfolio positioning.
If the objective of the forecast requires a chain of predictions, each step reduces the probability of success, often very materially. It is worthwhile bearing this in mind when presented with multi-step arguments, even if the analysis at each step is superb. A compelling single step argument may be less intellectually impressive for its lack of acrobatics, but a simple yet overwhelmingly strong insight is worth much more to investment outcomes. We will provide some examples later.
Inputs cannot be reliably measured: Economic data is released every working day and the implications are often expressed in terms of the impact on the outlook for GDP.
Despite its importance, GDP is difficult to calculate and is released around 2 months after the end of each quarter. However, this figure continues to be revised for 4 subsequent years. The following chart is supplied by the RBA and illustrates that the initial release, whose components attract a great deal of attention in tactical positioning, is generally very inaccurate. The error bands for the first release are almost as wide as the range of quarterly GDP outcomes, which is another way of saying the official GDP figure produced each quarter is only a rough but best available guess which will be tidied up over the course of the coming economic cycle.
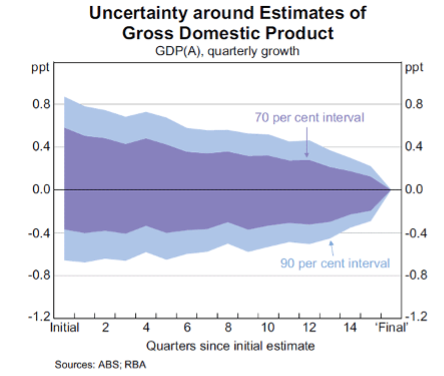
The forecasters of Australian GDP are engaged in something very close to forecasting a random figure, and these forecasts are even revised materially right up to the week before the actual figure is released as partial indicators are progressively reported by the Australian Bureau of Statistics. We hardly know where we are.
It is appropriate to be skeptical of a tactical investment process that relies upon producing superior estimates of GDP under these circumstances. Economic forecasting for the purposes of tactical investment positioning is a dreadful profession when the odds are inherently stacked so firmly against it. Should it be surprising that the accuracy of economic forecasts for GDP is so low when the subject of the forecast cannot be reliably measured? Anyone purporting to possess high accuracy in making forecasts of GDP or key sub-components should have their claims considered in light of this inconvenience.
Unsettled reaction functions: If the forecast variable is the outcome of a decision-making process that is opaque or somewhat unstable, accuracy will be impaired.
For example, the current orthodoxy is for central bankers to be relatively transparent in their thinking. In the US, various members of the FOMC regularly communicate their thoughts through the media, speeches, minutes, publications, testimony and also via official reports which compile their projected cash paths. These frequently reveal a diversity of thought. In Australia, the task of communication is generally left to senior RBA officials. It is generally believed that transparency helps to communicate the ‘reaction function’ of how the central bank will react to developments.
Unfortunately for forecasters, these reaction functions contain material uncertainties and frequently evolve. For example, just six months ago, the RBA assigned a very low likelihood that wage inflation would reach thresholds that would be consistent with raising rates for several years. They even anchored the yield on the April 2024 Australian Commonwealth Government bond to 0.1% for emphasis. However, now that inflation readings have been much stronger than anticipated, their most recent thinking is that underlying inflation will only fall to the top of the target inflation band in 2024!
The focus of their comments had been on the Wage Price Index measure of inflation and they emphasized a requirement that actual wage inflation would need to be observed to be above target levels for a while and not just projected. The definition of wage inflation has been subsequently broadened to include indicators like the Aggregate Earnings from the National Accounts and has evolved to even consider changes in the psychology of employers as a threshold matter. Most recently, it seems that only a single strong print of labour force costs will be sufficient to begin raising rates. The reaction function communicated to the public has clearly evolved in material ways.
Even if you could generate a reasonably accurate forecast of the future state of the economy, the central bank reaction functions are obviously somewhat unstable. A range of plausible and reasonable reaction functions exist across central banks and, indeed, within each of the decision-making committees. This type of uncertainty in decision rules also permeates government budget policies, geopolitical developments and even the economy is said to be driven by ‘animal spirits’ to an extent. As reaction functions of various kinds drive the political economy, and are inherently unstable or cannot be fully examined, there are genuine limits to the accuracy of forecasts which can be achieved.
Adaptive Processes: Financial market participants are consistently attempting to improve their outcomes and are motivated to improve their abilities. To the extent an opportunity exists to profit from projections, participants will move to position themselves to benefit from any perceived mispricing. In doing so, with respect to cash rates, the price of related bonds, futures and swaps will continually adjust to discount the collective best estimate.
The constant adaptation of market participants to these ends makes it very difficult to sustain an analytical advantage and generally serves to prevent egregious mispricing from persisting for long.
The aim of this example is to highlight that there is a high level of impenetrable uncertainty associated with forecasting if it involves characteristics of this nature.
The uncertainty associated with this line of enquiry generally cannot be materially overcome and this will irreparably impair the forecasting power of even the most brilliant analysts. Risk exposure associated with this type of complex decision should consequently be well contained if possible.
Consequences of overconfidence in complex decisions
If portfolio managers act as if their forecasts are more accurate than they are, the effect is not costless. They will tend to over-trade and produce portfolios that are overly concentrated but lack sufficient forecasting advantage to justify it. This produces portfolios that are excessively risky, and this reduces long term compound returns. Overall, engaging in this activity lowers risk-adjusted returns.
To illustrate, let us say the volatility of the S&P500 is 15% per annum and cash rates are zero. A long-term passive investor chooses to invest fully into the index and does nothing else. An extremely active investor, driven by strong but entirely unfounded convictions in their forecasts, attempts to outperform the passive alternative by spending all of the time either at 0% exposure or 200% exposure but maintains an overall average exposure of 100% over the longer term as well.
Although the average exposure to the index is identical in both instances, the active portfolio is riskier as the potential range of daily returns is wider given exposures can reach 200%. The expected volatility of the active portfolio would be 21% in this instance, which is materially higher than the passive alternative of 15%.
When a 50% loss is taken on an investment, it requires a 100% subsequent return to restore wealth to the original position. The larger the loss, the even greater the subsequent return required to restore it. This is known as the volatility drag effect. If two portfolios have equal expected returns on any given day, the compound return for a more volatile portfolio falls below that of the more stable one for these reasons.
Returning to our example, on any single day taken at random, the expected returns from the passive and active portfolios are identical. However, the losses experienced by the active portfolio are expected to be larger, and this requires a higher future average return to compensate for the higher volatility drag effect. The performance drag to a portfolio, compounded over the longer term, from the excess volatility of the active portfolio relative to the passive alternative in this example is worth approximately 1.1% per annum. This is a very meaningful figure over time and illustrates that a forecast advantage is required to concentrate risk in portfolios. In this instance, the active investor needs to be able to forecast the future direction of the S&P500 with an accuracy of 54% just to break-even with the passive alternative, and that is before considering matters like transaction costs, trading frictions and taxes. As we outlined earlier, that magnitude of forecasting advantage is hard to attain and merely represents a break-even point in this illustration.
This example is deliberately extreme to illustrate that there is a cost to wealth from misaligning true forecast power and portfolio settings.
Overconfidence is costly and we have hopefully illustrated that a portfolio of complex decisions will struggle to produce adequate forecasting advantages to justify large active positions developed on this basis.
Simpler Decisions can be powerful
An example of a simpler decision is a relative value comparison between the yields or spreads on substantively similar credit securities issued by the same company, albeit in different currencies. The following chart shows the credit spread issued by Westpac for hybrids in AUD and USD and illustrates that the relationship generally overlaps, came apart, and then reverted from extremes more recently:
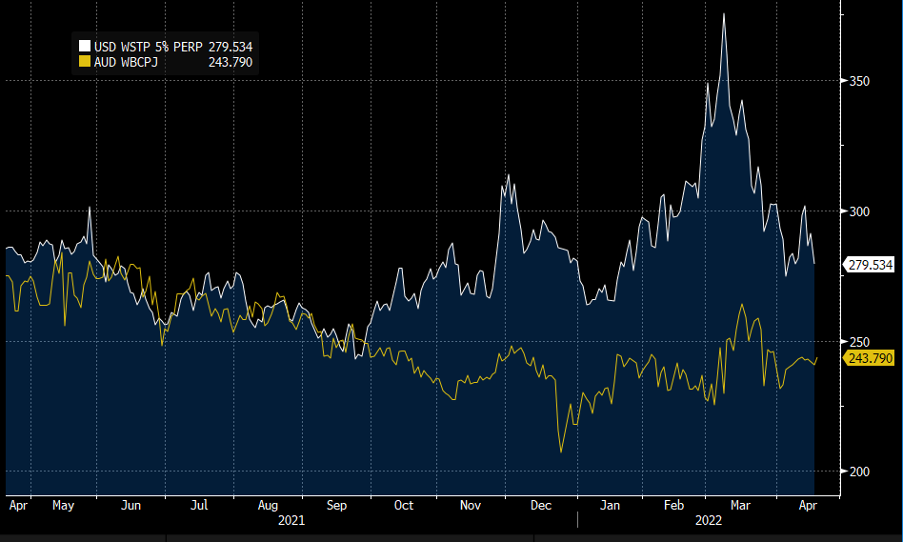
Acknowledging that the particulars of the securities are not fully identical, the gap between the two spreads is a form of mispricing, particularly when the gap between them becomes large. Unlike the prior examples of a complex decision, the extent of mispricing is:
- Fairly linear: The larger the gap, the greater the mispricing in an approximately 1:1 relationship;
- Requires a short inference chain: The extent of observed divergence translates directly into a mispricing estimate;
- Can be accurately measured: The price information is tradeable and not subject to measurement errors and subsequent revision;
- Does not rely materially on institutional decision rules: Over time, these types of relative value relationships tend to converge with high likelihood and this is often not particularly reliant on the vagaries of dominant decision-makers; and
- Is subject to slow-moving adaptive processes: The forces which drive the spreads together are present, but don’t operate too quickly. This may occur because the participants in the AUD and USD markets are different in composition and focus on different things. As a result, arbitrage activity is limited.
It should be evident that this type of relative value decision is far higher quality than a complex one.
Another example relates to the differences in the credit spread for RMBS which have been issued as a public security relative to the spreads on offer for similarly rated exposures held in warehouses. A warehouse is essentially a trust where pools of many mortgages are built up before being structured and sold as a traditional RMBS. The underlying collateral is hence substantively similar in nature.
In a recent transaction, the spreads on offer for the BBB-rated tranche of a block of mortgages being developed in a warehouse was 6% per annum. When the block of mortgages was compiled and sold in public form, the BBB-rated tranche offered a spread of 3%. The difference largely relates to a premium for illiquidity and the complexity associated with negotiating wholesale loan agreements. There are other differences as well relating to the expected term of the security and other creditor rights available when lending to a warehouse.
Given the underlying collateral for these assets can be very similar, decisions comparing the relative spread between public RMBS and private warehouse exposures are quite straightforward. We are then able to ascertain whether we are happy with the additional premium available from warehouse exposures with considerable clarity.
Where the ability exists to extract returns from these simpler opportunities, which includes consideration of matters like product design, investment time horizon and capacity management, they tend to offer far better risk-adjusted outcomes.
Calibration of portfolio exposures
Portfolio construction consists of many different elements. Even though forecasting absolute bond yields via macro analysis may be complex, this does not imply that bonds have no place in a portfolio. Bonds may provide ballast, regular income and help match liability streams. The same applies to any strategic asset class exposure where their characteristics earn them an allocation.
The key assertion of this note is that the risk arising from attempting to tactically allocate to bonds (or other assets) on the basis of economic forecasts or other complex insights is very difficult in many, but not all, circumstances. The uncertainties are often materially irreducible, even if the analysis may seem inspired. Where simpler decisions of the type we have illustrated are available, these should generally consume a much larger portion of the tactical risk budget.
Realm's approach
The concepts in this note are applied to our portfolios as appropriate. Not all portfolios invest in warehouse exposures or hold corporate debt, for example. However, we limit our exposure to risks arising from complex decisions where possible and are acutely aware of the frictions associated with misaligning forecasting ability and portfolio risk allocation.
Most recently, these disciplines helped to prevent the Realm High Income Fund from incurring greater losses as bond markets sold off strongly. As with many economic forecasters, since November last year, we felt that the RBA would have to raise rates ahead of their guidance at the time. However, markets had moved well ahead of our expectations, largely tracking the US inferred rate path despite very different economic circumstances at the time. This seemed too steep a rate path and we believed that (shorter dated) bonds would subsequently rally.
Instead, despite experiencing an Omicron wave over the turn of the year, the strength of the economic recovery exceeded our expectations and the Ukraine war pushed inflation even higher despite supply chain issues reaching a temporary peak beforehand. Lockdowns in Shanghai and Beijing further exacerbated these issues. As mentioned previously, the RBA’s reaction function also continued to evolve over this period and lowered the pivotal threshold relating wages growth. Ultimately, yields on bonds and bank bill swaps kept rising.
Whilst our expectations did not come to fruition on this occasion, we recognized that this was a complex decision, would have low predictive value despite being a strong consensus position, and we limited our portfolio exposure to this idea. Our positioning also considered the balance of risks from bonds relative to other asset classes.
Importantly, our disciplines remained in place despite credit spreads being compressed to such a low level that we could not generate forward-looking returns in line with portfolio objectives if conditions were to remain unchanged. In those circumstances, the increasing term premium in bonds was especially enticing. However, we did not build large tactical positions in bonds and we did not reach for complex decisions in credit either. This prevented us from materially overweighting bonds too early in the sell-off as the Delta wave receded and engaging in yield-seeking behaviours when markets were most expensive.
Realm is your partner
Our clients are our lifeblood. With deep experience investing in Australian Credit and Fixed Income markets, Realm is result-oriented. We are always focused on delivering client outcomes. Click the 'FOLLOW' button below to receive our insights.
2 funds mentioned